mvpa2.clfs.warehouseΒΆ
Collection of classifiers to ease the exploration.
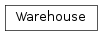
Functions
absolute_features () |
Returns a mapper that converts features into absolute values. |
is_sequence_type |
isSequenceType(a) – Return True if a has a sequence type, False otherwise. |
maxofabs_sample () |
Returns a mapper that finds max of absolute values of all samples. |
Classes
BLR ([sigma_p, sigma_noise]) |
Bayesian Linear Regression (BLR). |
FeatureSelectionClassifier (clf, mapper, \*\*kwargs) |
This is nothing but a MappedClassifier . |
FixedNElementTailSelector (nelements, \*\*kwargs) |
Given a sequence, provide set of IDs for a fixed number of to be selected elements. |
FractionTailSelector (felements, \*\*kwargs) |
Given a sequence, provide Ids for a fraction of elements |
GNB (\*\*kwargs) |
Gaussian Naive Bayes Classifier . |
GPR ([kernel]) |
Gaussian Process Regression (GPR). |
GeneralizedLinearKernel (\*args, \*\*kwargs) |
The linear kernel class. |
LDA (\*\*kwargs) |
Linear Discriminant Analysis. |
LinearCSVMC ([C]) |
C-SVM classifier using linear kernel. |
LinearKernel (\*args, \*\*kwargs) |
Simple linear kernel: K(a,b) = a*b.T |
LinearLSKernel (\*args, \*\*kwargs) |
A simple Linear kernel: K(a,b) = a*b.T |
LinearNuSVMC ([nu]) |
Nu-SVM classifier using linear kernel. |
LinearSVMKernel |
alias of LinearLSKernel |
MulticlassClassifier (clf[, bclf_type]) |
Perform multiclass classification using a list of binary classifiers. |
OddEvenPartitioner ([usevalues]) |
Create odd and even partitions based on a sample attribute. |
OneWayAnova ([space]) |
FeaturewiseMeasure that performs a univariate ANOVA. |
PLR ([lm, criterion, reduced, maxiter]) |
Penalized logistic regression Classifier . |
PolyLSKernel (\*\*kwargs) |
Polynomial kernel: K(a,b) = (gamma*a*b.T + coef0)**degree |
QDA (\*\*kwargs) |
Quadratic Discriminant Analysis. |
RandomClassifier (\*\*kwargs) |
Dummy classifier deciding on labels absolutely randomly |
RangeElementSelector ([lower, upper, ...]) |
Select elements based on specified range of values |
RbfCSVMC ([C]) |
C-SVM classifier using a radial basis function kernel |
RbfLSKernel (\*\*kwargs) |
Radial Basis Function kernel (aka Gaussian): |
RbfNuSVMC ([nu]) |
Nu-SVM classifier using a radial basis function kernel |
RbfSVMKernel |
alias of RbfLSKernel |
RegressionAsClassifier (clf[, centroids, ...]) |
Allows to use arbitrary regression for classification. |
SMLR (\*\*kwargs) |
Sparse Multinomial Logistic Regression Classifier . |
SMLRWeights (clf[, force_train]) |
SensitivityAnalyzer that reports the weights SMLR trained |
SVM (\*\*kwargs) |
Support Vector Machine Classifier. |
SensitivityBasedFeatureSelection (...[, ...]) |
Feature elimination. |
SigmoidLSKernel (\*\*kwargs) |
Sigmoid kernel: K(a,b) = tanh(gamma*a*b.T + coef0) |
SplitClassifier (clf[, partitioner, splitter]) |
BoostedClassifier to work on splits of the data |
SplitRFE (lrn, partitioner, fselector[, ...]) |
RFE with the nested cross-validation to estimate optimal number of features. |
SquaredExponentialKernel ([length_scale, sigma_f]) |
The Squared Exponential kernel class. |
Warehouse ([known_tags, matches]) |
Class to keep known instantiated classifiers |
kNN ([k, dfx, voting]) |
k-Nearest-Neighbour classifier. |